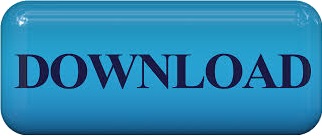
For a country or an area, sufficient COVID-19 tests can greatly decrease the number of unconfirmed infections, reduce the speed of disease transmission, and help in an accurate trend analysis to evaluate the pandemic situation. The population movement of a large group of presymptomatic and asymptomatic patients is a key factor contributing to the spread of COVID-19. Confirmed COVID-19 cases are mostly quarantined or self-isolated, while presymptomatic and asymptomatic patients form a large group of unregistered patients, who can move freely and infect close contacts easily. Presymptomatic and asymptomatic patients play a key role in the spread of COVID-19. The tremendous number of COVID-19 cases may be attributable to multiple factors.

As a result, the World Health Organization (WHO) set COVID-19 to the highest crisis alert level by declaring the COVID-19 outbreak a global pandemic. A total of 34488636 COVID-19 cases and 1026176 deaths were reported worldwide and more than 60% of the global population went into coronavirus lockdown. By September 30, 2020, more than 200 countries had been affected, with major outbreaks in the United States, India, Brazil, Russia, Colombia, Peru, and others. Although most countries launched emergency responses early in the outbreak, the COVID-19 still swiftly spread from metropolitan areas to urban areas, from countries to countries. However, at present, there is no unified scientific conclusion on the origin of SARS-CoV-2. Evolutionary virologist found bats may be the origins of SARS-CoV-2, which has a long evolutionary history. The outbreak of COVID-19 has progressed with a tremendous impact on the economic, social behavior, environment, climate, etc. This new infectious disease spread worldwide in less than half a year in 2020, causing devastation to the human population. The novel coronavirus disease 2019 (COVID-19), caused by severe acute respiratory syndrome coronavirus 2 (SARS-CoV-2), has high transmissibility. Hence, the proposed model demonstrates superior predictive power over other benchmark models. ), median absolute error (MAD), and mean absolute percentage error (MAPE) than other models. ), adjusted coefficient of determination ( nearest neighbors (KNN), decision tree (DT), adaptive boosting (Ada), RF, gradient boosting DT (GBDT), support vector regression (SVR), extra trees (ETs) regressor, CatBoost (CAT), LightGBM (LGB), XGBoost (XGB), and BLS.The RF-Bagging BLS model showed better forecasting performance in terms of relative mean-square error (RMSE), coefficient of determination ( In addition, we compared the forecasting results with linear regression (LR) model, Then, we combine the bagging strategy and BLS to develop a random-forest-bagging BLS (RF-Bagging-BLS) approach to forecast the trend of the COVID-19 pandemic. Here, we leveraged random forest (RF) to screen out the key features. We proposed a machine learning model for COVID-19 prediction based on the broad learning system (BLS).

In this work, a large COVID-19 data set consisting of COVID-19 pandemic, COVID-19 testing capacity, economic level, demographic information, and geographic location data in 184 countries and 1241 areas from December 18, 2019, to September 30, 2020, were developed from public reports released by national health authorities and bureau of statistics. Recently, the analysis and forecast of the COVID-19 pandemic have attracted worldwide attention. The rapid geographic spread of COVID-19, to which various factors may have contributed, has caused a global health crisis.
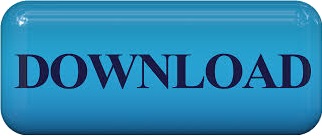